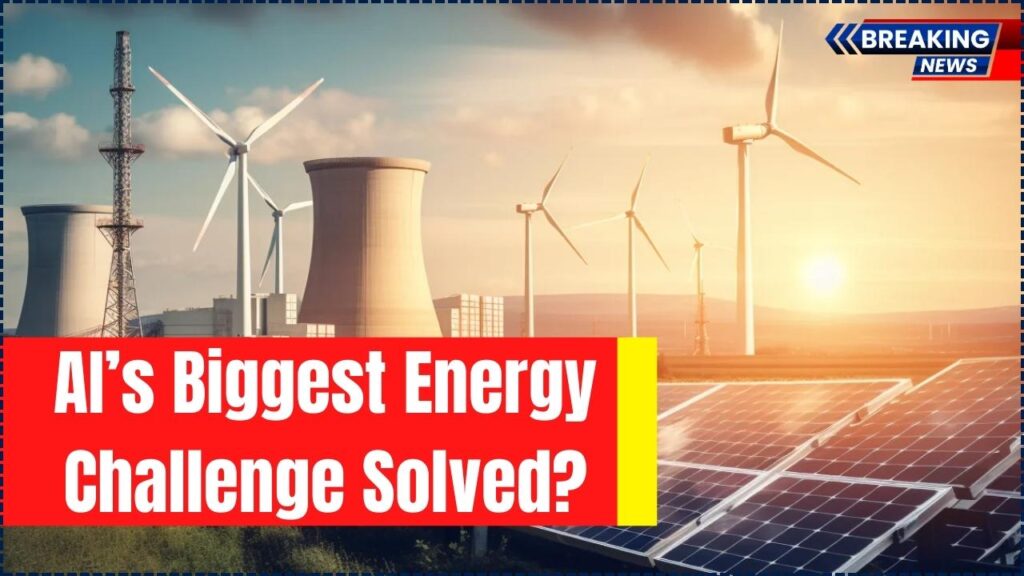
AI’s Biggest Energy Challenge Solved: Artificial intelligence (AI) is transforming industries worldwide, from healthcare to finance and beyond. However, one of the biggest challenges facing AI today is its massive energy consumption. Training and running AI models, especially large-scale neural networks, requires enormous computing power, leading to high electricity costs and environmental concerns.
Recent innovations in magnetic chip technology may provide a breakthrough solution. Scientists and engineers are developing energy-efficient AI chips that could significantly reduce power usage while maintaining high processing speeds. Could this be the future of sustainable AI? Let’s explore.
AI’s Biggest Energy Challenge Solved
Breakthrough | Impact on AI Energy Consumption | Potential Benefits |
---|---|---|
Magnetic AI Chips | Reduce energy usage by up to six times | Lower operating costs and improved efficiency |
Spintronic Technology | Uses electron spin instead of charge for computing | Minimizes power loss and heat generation |
Neuromorphic Computing | Mimics human brain processes | More efficient AI model training and deployment |
Integration of Memory & Processing | Eliminates data transfer bottlenecks | Faster and more eco-friendly AI systems |
AI’s energy problem is a growing concern, but magnetic chip technology could be the breakthrough needed for sustainable AI development. By reducing energy consumption while maintaining high performance, spintronic AI chips and neuromorphic computing could revolutionize the future of artificial intelligence.
As researchers continue to improve these technologies, we may soon see AI models running on ultra-efficient, low-power hardware, making AI more accessible, affordable, and environmentally friendly.
1. The AI Energy Crisis – Why It Matters
The rapid advancement of AI has come with a heavy energy cost. According to a study by the University of Massachusetts Amherst, training a single large AI model like GPT-4 consumes as much energy as five cars over their entire lifetimes. As AI adoption grows, power demands continue to skyrocket.
Why is AI so Energy-Intensive?
- Large-scale data processing requires vast amounts of computational resources.
- Deep learning models demand multiple iterations and adjustments before deployment.
- Traditional semiconductor chips (such as GPUs and TPUs) rely on electrical charge, leading to energy loss as heat.
This situation has prompted researchers to find alternatives that can drastically cut AI’s energy consumption while maintaining performance.
2. How Magnetic AI Chips Work – The Science Behind the Breakthrough
What Are Magnetic AI Chips?
Magnetic AI chips, also known as spintronic chips, use electron spin instead of charge to perform calculations. Unlike conventional chips, which rely on electrical currents (causing heat and energy waste), these chips use magnetic properties to store and process information with far less power loss.
Key Advantages of Magnetic Chips:
- Lower Power Consumption: Spintronic chips use minimal energy compared to conventional silicon-based processors.
- Reduced Heat Generation: Less energy is wasted as heat, making AI systems more efficient.
- Faster Processing Speeds: Magnetic chips enable faster data transfer and eliminate bottlenecks.
Example: Researchers from Tohoku University and Japan’s National Institute for Materials Science have developed a new spintronic device that integrates magnetic and electrical properties, offering a highly efficient AI processing alternative.
3. AI’s Future: Neuromorphic Computing & Brain-Like Processors
Neuromorphic computing is another game-changing technology in AI energy efficiency. It mimics the human brain’s neural networks, allowing for adaptive learning with significantly lower power consumption.
How It Works:
- Uses artificial neurons and synapses to process information similarly to a biological brain.
- Eliminates the need for large data centers, reducing power consumption.
- Can operate on low-power devices, such as smartphones and IoT devices.
Example: Oregon State University recently developed an AI chip that improves energy efficiency by six times, bringing AI processing closer to neuromorphic efficiency.
4. Magnetic Chips vs. Traditional AI Chips – A Comparative Analysis
Feature | Traditional AI Chips (GPUs/TPUs) | Magnetic AI Chips |
---|---|---|
Power Consumption | High (1000s of watts per model) | Low (significantly reduced) |
Processing Speed | Slower due to data transfer bottlenecks | Faster due to integrated memory & processing |
Heat Generation | High | Minimal |
Cost Efficiency | Expensive due to high power use | More cost-effective long-term |
Environmental Impact | High carbon footprint | Lower carbon emissions |
Source: WSJ Tech
Gemini 2.0 Flash: Google’s Latest AI Model That Can ‘Think’ – Full Details!
ChatGPT’s Web Search Just Got Easier! OpenAI Removes Login Requirement
Qwen 2.5 AI by Alibaba Cloud: The AI Model That Could Replace ChatGPT and DeepSeek
FAQs On AI’s Biggest Energy Challenge Solved
1. How much energy do AI models currently consume?
Training large AI models can consume hundreds of megawatt-hours, equivalent to powering a small city for weeks.
2. Are magnetic AI chips available for commercial use?
Currently, magnetic chips are in the research and development phase, but major tech companies are actively exploring their applications.
3. How do magnetic chips compare to quantum computing?
While quantum computing is still experimental, magnetic AI chips are closer to commercialization, offering a practical energy-efficient solution for AI.
4. Which companies are leading AI energy-efficiency research?
Companies like IBM, Intel, and Nvidia, along with universities such as MIT and Stanford, are leading efforts in energy-efficient AI chip design.
5. How soon will we see these chips in AI applications?
Experts predict that within 3-5 years, we will see energy-efficient AI chips being integrated into mainstream computing and AI training centers.